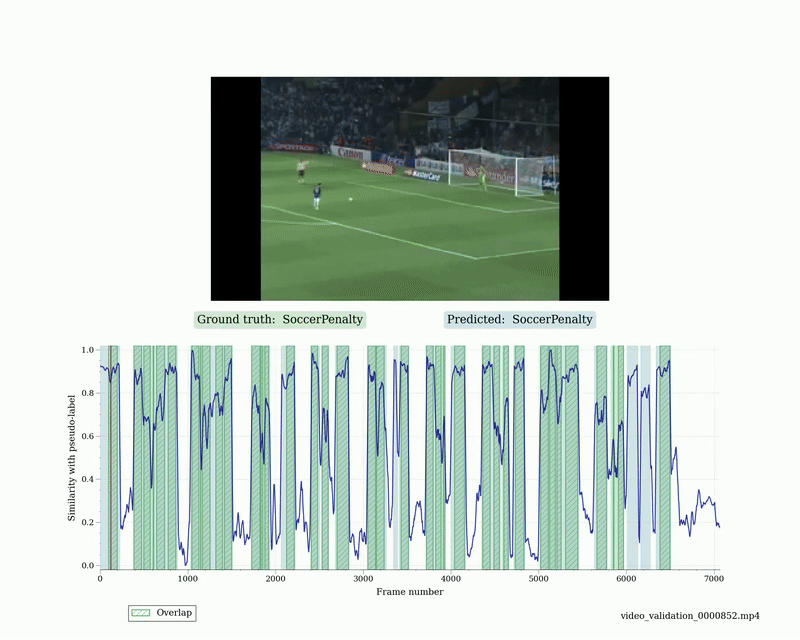
Temporal Action Localization (TAL) seeks to identify and locate actions in untrimmed videos. While effective, training-based zero-shot TAL approaches assume the availability of labeled data for supervised learning, which can be impractical in real-world applications. Furthermore, the training process naturally induces a domain bias into the learned model, which may adversely affect the model’s generalization ability to arbitrary videos. We have developed T3AL, a method that addresses TAL without requiring the collection of training data. T3AL leverages a pre-trained vision and language model and adapts it at test-time on a stream of unlabelled videos without prior supervised training.