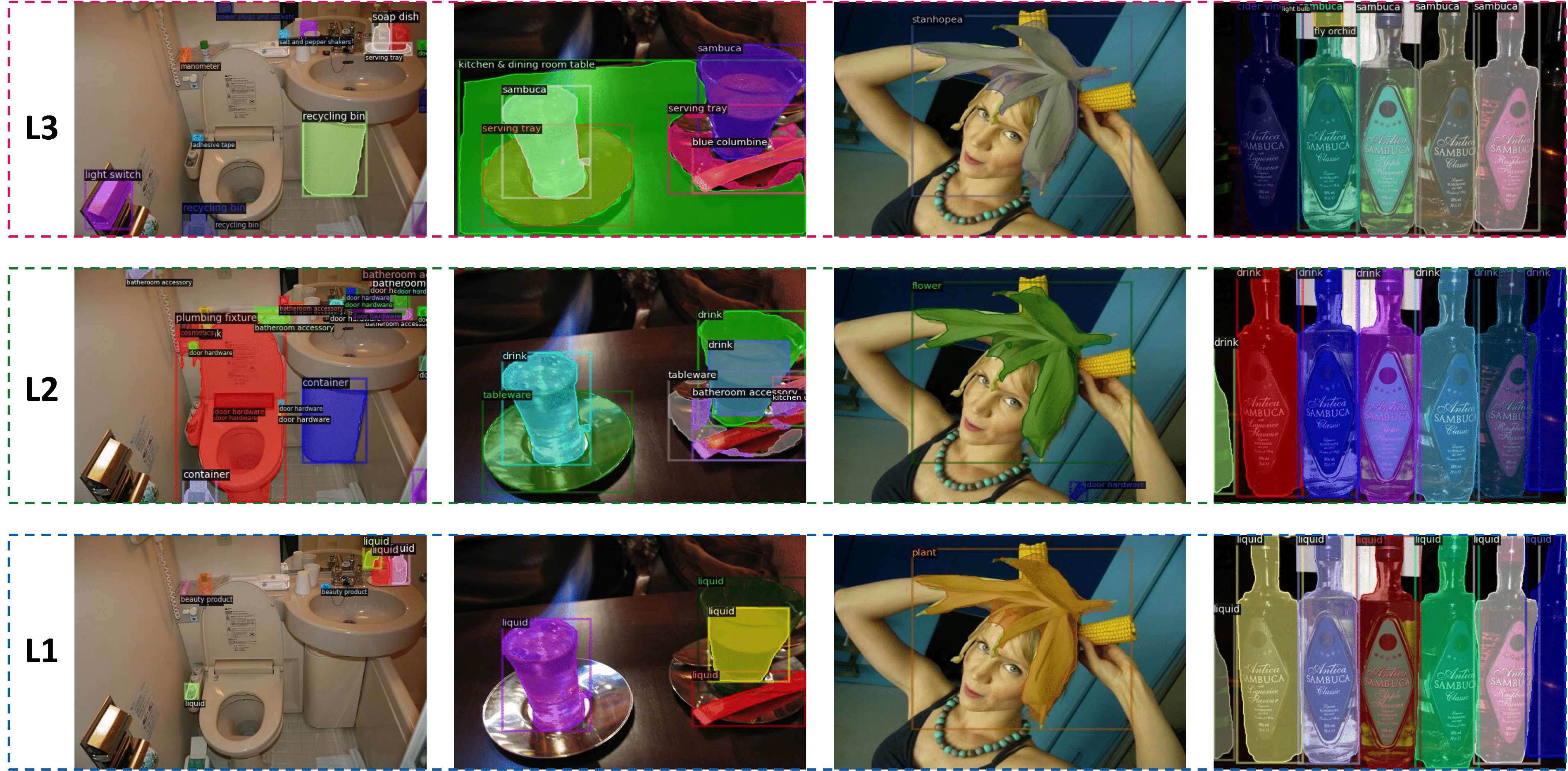
Open-vocabulary object detection (OvOD) models have become increasingly practical due to their flexibility. These models allow users to specify a set of class names to detect in a zero-shot manner during deployment. However, users might define the class names with varying levels of semantic granularity based on their preferences, causing fluctuations in the models’ performance. To address this issue, our project leverages semantic hierarchy to enhance the robustness and performance of OvOD models, ensuring they perform consistently regardless of the granularity specified by the users.