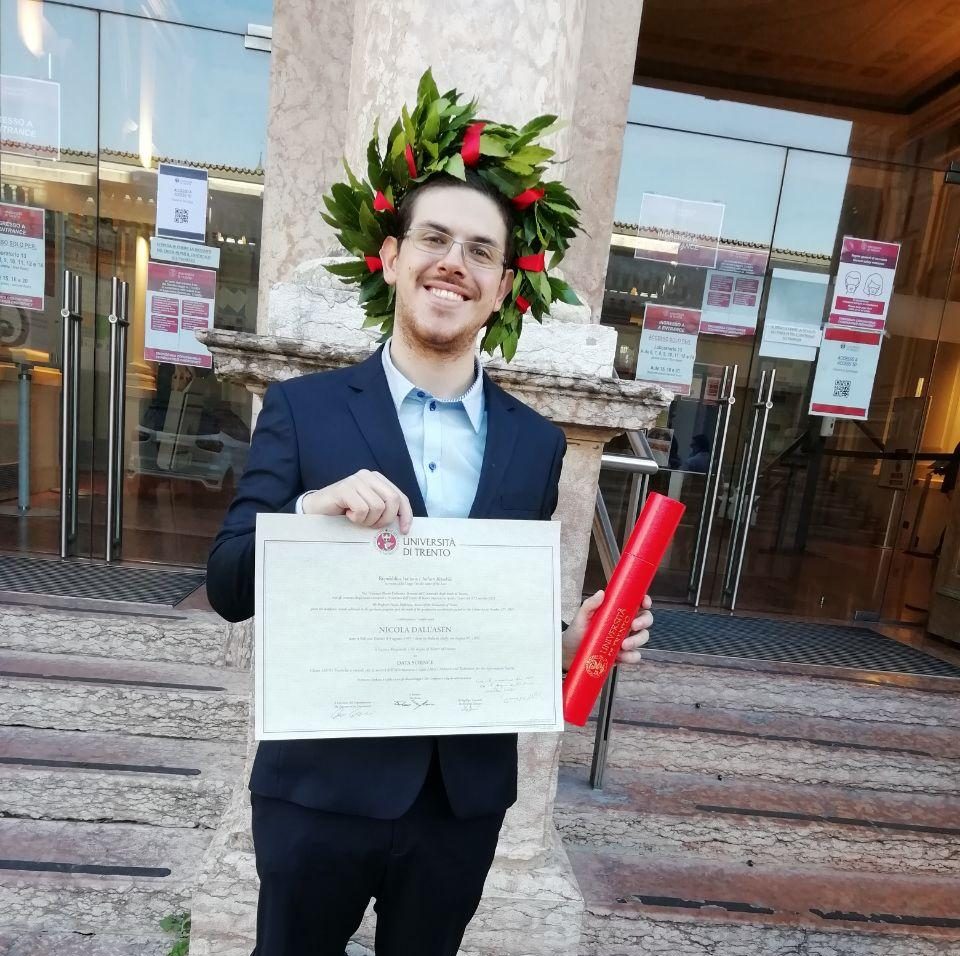
We are glad to announce that Nicola, our ex-intern student, has been shortlisted for the Thesis Award in Trento! With the goal to foster a closer and more constructive relationship among citizens, the municipality and University, the Municipality of Trento awarded thesis are of interests for the development and innovation of the territory, providing a space for public presentations of the works.
Nicola joined DVL unit as an intern in March 2021. His hard work and commitments not only contributed to the technological development of video anonymisation under the MARVEL project, but also led to a conference publication and an outstanding thesis titled Anonygan: a pose-preserving graph-based generative face anonymisation framework.
Nicola’s thesis discusses a growing concern, the people’s privacy. In Europe, the legislation is going towards an always increasing protection of personal data and limitations on their use. This protection can be achieved via redaction of personally identifiable information (PII) of a data subject. Classical techniques can completely remove this information hindering further analyses, e.g. sentiment analysis or anomaly detection. The use of Deep Learning and in particular of Generative Models can achieve both de-identification and analyses preservation. In the task of face anonymisation, state-of-the-art methods focus on the naturalness of the generated images and do not take into explicit consideration the elements that preserve the analysability of a face, i.e. expression and pose.
After an extensive exposition of the legal framework regulating this task on the European territory and an analysis of related works and their limitations, together with Nicola, we propose AnonyGAN, a GAN-based solution for face anonymisation which replaces the visual information corresponding to a source identity with a condition identity provided as any single image. With the goal to maintain the geometric attributes of the source face, i.e., the facial pose and expression, and to promote more natural face generation, we propose to exploit a Bipartite Graph to explicitly model the relations between the facial landmarks of the source identity and the ones of the condition identity through a deep model. We further propose a landmark attention model to relax the manual selection of facial landmarks, allowing the network to weight the landmarks for the best visual naturalness and pose preservation. Finally, to facilitate the appearance learning, we propose a hybrid training strategy to address the challenge caused by the lack of direct pixel-level supervision. We evaluate our method and its variants on two public datasets, CelebA and LFW, in terms of visual naturalness, facial pose preservation and of its impacts on face detection and re-identification. We prove that AnonyGAN significantly outperforms the state-of-the-art methods in terms of visual naturalness, face detection and pose preservation.